Anticipate
Augmenting thought processes by uncovering hidden assumptions through AI
Niklas MuhsSummary
This idea envisions a future where Artificial Intelligence (AI), particularly Large Language Models (LLMs), augments rather than automates design and decision-making. Rather than simplifying away complexity, it explores how AI can help designers and decision-makers navigate uncertainty and make more informed decisions.
At its core is the challenge of addressing “unknown unknowns” — factors that are not immediately apparent but can significantly impact outcomes. By leveraging AI's capabilities, designers can stimulate reflection, challenging their own mental models, biases, and assumptions, and explore a broader spectrum of possibilities, leading to more robust and insightful solutions. This preserves the richness of human creativity while opening new avenues for thoughtful, inclusive, and resilient design.
anticipate promotes a dynamic, collaborative design environment where human agency is enhanced, not eroded — where systems augment rather than automate, support rather than steer, and question rather than conform. It points toward a future where AI and human creativity work in concert to foster more thoughtful, nuanced, and ethical design practice.
The exploration underscored the importance of maintaining human agency in the age of artificial intelligence, advocating for systems that augment rather than automate, that question rather than conform. As we stand at the intersection of technological advancement and human-centered design, I believe the path forward is one of collaboration, critical thinking, and continuous reflection. Niklas Muhs
Key Concepts
- AI as creative amplifier AI should be used as a tool to enhance human creativity — not to replace it, but to expand its reach and potential.
- Bias-aware design Designers’ mental models and biases shape how they approach uncertainty. AI can help surface and challenge these patterns, opening space for more objective and innovative solutions.
- Surfacing the unknown AI can augment the design process by stimulating reflection, questioning assumptions, and helping uncover the elusive “unknown unknowns” that often shape outcomes.
- Managed complexity The goal isn’t to simplify design but to manage its complexity. With AI as a thinking partner, designers can navigate ambiguity with greater confidence — leading to deeper, more resilient results.
anticipate offers a new paradigm for AI-assisted design — one that centers human judgment, embraces complexity, and leverages machine intelligence to deepen, not dilute, our creative and strategic thinking. It challenges us to build systems that illuminate uncertainty, foster reflection, and support more thoughtful, inclusive, and resilient decision-making.
This idea was originally developed as a bachelor thesis by Niklas Muhs, and is presented alongside a prototype and project materials at anticipate.studio. The prototype can be explored at prototype.anticipate.studio.
Introduction
The content of the following article emerged from my bachelor thesis and examines the integration of artificial intelligence, particularly Large Language Models, into the ideation and design process as a form of augmentation. Recognizing the significant role of design in navigating socio-technological changes, the study investigates how AI can support designers and decision-makers in addressing uncertainties. The complex nature of the design process, encompassing problem identification, framing, redefinition, and solution creation, is underscored.
The ability of a designer or decision-maker to manage uncertainties depends on mental models, biases, and the capacity to tackle the “unknown unknowns.” The article aims to reveal that designers as well as AI can inadvertently perpetuate societal thought structures, which may limit its efficacy in solving problems that demand radical shifts in thinking. However, I posit that AI can augment the design process by stimulating designers’ reflection and helping them to challenge their assumptions and biases. This augmentation preserves the design process’s complexity while mitigating the limitations of fixed thinking patterns.
This article identifies the potential and challenges of incorporating AI into the design process. It advocates for the responsible use of AI to enhance design, fostering innovation while critically engaging with ethical considerations. The study thus aims to contribute to a growing field and is directed towards individuals involved in the design or operating in uncertain environments, urging them to view design as an essential facilitator of innovative️ solutions in combination with the cautious integration of AI.️
Artificial intelligence
Prominent artificial intelligence architectures can be divided into two broad categories, among others: generative and discriminative. Generative AI systems produce new artifacts and can provide different outputs for the same input. In contrast, discriminatory AI systems focus on determining labels, classifications, and decisions and aspire to deterministic outcomes.
Computers operate based on algorithms provided by humans. Conversely, AI leverages statistical learning methods to achieve desired outcomes by examining large amounts of data for potential patterns. This behavior is beneficial when the complexity of the desired results makes it difficult to codify the rules algorithmically because of large amounts of data or complex interrelationships.
While the emergence of capabilities such as reasoning increases with the scale of models, the ability to track these processes becomes more difficult. In Interviews, it becomes apparent that even researchers at OpenAI are often surprised by the results and are continually investigating how much awareness AI systems have developed.
Since I was primarily interested in the ability of AI to support reasoning in design processes, I was particularly interested in Large Language Models because of their emerging reasoning capabilities. For the remainder of this work, I will refer to the term “artificial intelligence” as Large Language Models unless specified otherwise.
AI ethics
Transitioning from the technical aspects to ethical considerations, it’s crucial to recognize the profound impact that AI can have on society. As we delve into the ethics of AI in design, we must consider how these technologies align with our moral values and societal norms.
Implementing AI in design brings a host of ethical considerations and challenges that need to be addressed. A responsible integration of AI in design should adhere to eight essential criteria defined by the IEEE as shown in the illustration above.
Engenhart and Löwe point out that AI systems are inherently biased as they cannot accurately represent reality but make predictions based on their data inputs. This bias manifests in technical and socio-political distortions. The data employed in widely used AI models, such as those from OpenAI, typically represent a specific segment of global society and do not equally account for all aspects.
There is a limit to human participation in AI decision-making processes, and the methods through which AI systems arrive at conclusions often need to be made more transparent. These systems rely on the quality of their data, so biases in decision systems can amplify pre-existing prejudices within the data. Other ethical concerns include automation and employment, autonomous systems, machine ethics, artificial moral agents, and the concept of singularity.
It is crucial to note that AI is inherently discriminatory or biased due to the disparity between the environment and data and the discrepancy between data and statistical approximation. Therefore, while the application of AI in design can enhance the design process, we must be aware of these inherent biases and ethical considerations to ensure we use AI responsibly.
It is essential to reflect on how these biases and ethical concerns translate within the design process. This investigation highlights the need for a reevaluation of design’s role and purpose in the age of AI. By acknowledging the limitations and potential biases of AI, designers are prompted to reconsider not just the tools they use but also the fundamental approach to their work.
Design in uncertainty
Design has too often been deployed at the low-value end of the product spectrum, putting the lipstick on the pig Dan Hill
This quote was a guiding inspiration for the approach to design that this work is trying to investigate and augment. Furthermore, Dan Hill adds in his book Dark Matter & Trojan Horses:
In doing this, design has failed to make a case for its core value, which is addressing genuinely meaningful, genuinely knotty problems by convincingly articulating and delivering alternative ways of being.2 Dan Hill
In the spirit of the quote and the book, this article will address the part of the design that deals with knotted but significant problems. Therefore, this section explores how design frameworks and Design Thinking can support solving problems, especially in uncertainty.
To comprehend design and design processes more profoundly, we will turn to the definition offered by Herbert Simon in “The Sciences of the Artificial.” According to Herbert Simon,
Everyone designs who devises courses of action aimed at changing existing situations into preferred ones. […] Natural sciences deal with how things are; design deals with how things should be. Herbert Simon
These definitions are particularly relevant for this investigation as they weave together two significant concepts — the perception of the existing situation and the desirability of new conditions. They underscore the essence of design as a discipline that does not merely accept “what is” but ambitiously navigates toward “what should be.” Thus, by reframing our understanding of design, we can begin to value its power to influence and shape the world around us, embracing uncertainty as a platform for innovation rather than a limitation.
Innovation and design frameworks
In the following sections, different innovation and design frameworks are discussed, and their implications on uncertainty are considered. These will work as a foundation for the subsequent interviews with design professionals, which are being analyzed to provide insight into difficulties and complexities in applied design processes. Several design capabilities considered with uncertainty and AI emerged from the formative interviews.
Design Thinking
Design Thinking focuses on understanding human needs and reframing problems in human-centric ways. It encourages empathy, optimism, iteration, creative confidence, experimentation, and embracing ambiguity and failure. However, while impactful, it also has shortcomings.
As Don Norman wrote in his book “Design for a Better World”, Design work often fails to account for potential impacts on people or the environment. It is suggested that such design processes tend to neglect the possible waste products that could result and the overall effect on people’s lives.
In an interview with Dark Horse Innovation, Fabian Gampp, one of the co-founders of the System Mapping Academy, compares Design Thinking with systemic thinking, translated into English:
Unlike Design Thinking, system mapping is not (only) based on the needs of the users and does not per se claim to solve these needs. In applying system mapping, the focus is on the long-term health of the entire system. Fabian Gampp
This quote describes one of the shortcomings of the user-centered design approach, in focusing too much on the needs of specific user groups.
Consequently, there has been a movement within Design Thinking to broaden the scope of consideration. This shift is demonstrated through the progression from user-centered to human-centered and eventually to humanity-centered design.
Futures Thinking
As described by Groß and Mandir, Design Futuring is a methodology dedicated to exploring, creating, and negotiating future scenarios. It provides designers with techniques and conceptual tools to systematically and efficiently propose various future scenarios. This methodology goes beyond forecasting, encouraging creativity to work towards the development of more “preferable” futures. Design Futuring holds a dual impact; it expands the toolkit and domain of influence for designers and establishes a shift in the design discipline toward more strategic and creative thinking.
A particular model integral to Futures Thinking is the Futures Cone developed by Joseph Voros, which can be seen above. As Groß and Mandir put it, this isn’t about tracing a straight line into tomorrow but rather embracing a broad spectrum of what might unfold. The model can be imagined as standing in a snowfall with a flashlight; here, each falling snowflake symbolizes a potential future event, while the flashlight’s cone represents the spectrum of foreseeable future events. This analogy helps to comprehend that everything beyond the cone of light remains unknown, thereby uncertain. In the realm of Design Futuring, the Futures Cone serves as a model to categorize insights derived from exploring different future scenarios. The concept of the futures cone becomes relevant again in later considerations.
Nohr and Kaldrack, delve into the power of speculative thinking as a tool to shake up our usual ways of seeing the world. It involves transcending established knowledge paradigms and uncovering the concealed genealogies of the present to envision new futures. However, this exploration must acknowledge the entanglement of one’s perspective within power and knowledge regimes and an understanding of how they evolved, which emphasizes the necessity of critical reflection in futures thinking.
Larsen’s work in 2022 introduces us to the concept of “futures literacy,” a skill set that enables us to harness our assumptions about what comes next and use the unknown as a canvas for creativity. It is about recognizing assumptions about the future and converting uncertainty from planning into a resource. Individuals can identify and foster their capacity to shape and invent new anticipatory assumptions by imagining different futures. Shifting this anticipatory ability from an unconscious to a conscious state is crucial to becoming future literate.
Lean UX
Lean UX focuses on the design process, team collaboration, and organization. It takes inspiration from Eric Ries’ Lean Startup methodology, which encompasses a loop containing the “Build-Measure-Learn” cycle. Assumptions are vital in the Lean UX methodology and are being added to the Build-Measure-Learn cycle of Lean Startup. At the beginning of a project, speculations range from the obvious to the ones that become only apparent when it is too late as described by Gothelf and Seiden. This approach also necessitates finding and doubting these assumptions and validating them systematically and comprehensively.
The uncertainty inherent in startup environments makes the conscious formation of assumptions and the iterative Assumption-Build-Measure-Learn cycle is pivotal to Lean UX. Lean UX provides the required methods to drive and structure the appearance of beliefs, making it an effective tool for startups navigating uncertainty.
Design competencies
The following sections will explore various competencies essential to the design process. Understanding these skills can help understand how problems within the design process can be solved and how artificial intelligence could offer support within these problem-solving processes.
In his book “Design for a Better World,” Don Norman touches on a fascinating contradiction within design itself: its most significant flaw, and yet its strongest asset is that designers often start projects without any specific field expertise. While design needs to gain content expertise for every project, this absence of preconceived notions and prior knowledge empowers designers. Such a lack of assumptions prevents designers from suggesting solutions to problems prematurely, allowing them to explore various possibilities thoroughly.
Horst Rittel delves deeper into the complex nature of planning in the book “Thinking Design”. He argues that knowledge for a planning problem usually lies with the users rather than with the experts, and there are no real experts in solving complex issues except for the process of problem treatment.
Rittel further underscores the difficulty of addressing complex problems through scientific methods alone, framing the process of tackling wicked problems as an argumentative one that requires intuition and rationality. The quality of a plan (or solution) is determined by the certainty with which the goal is achieved while avoiding side effects and consequences.
He goes on to explain that a solution is a sequence of operations and manipulations that transform the current state into a desired one. This process of searching and constructing functions lies in the divergence and convergence of ideas. The implementation of plans always involves the use of resources and necessitates consideration of the irreversibility of plans. Models can help to assess the impact beforehand. They can range from sketches, cardboard, dynamic, and computer models to mental models, aiding this process. Models help make decisions more traceable and justifiable, fostering transparency in the planning process.
Integrating these perspectives, designers possess a comprehensive understanding of the creation process, which involves the ability to work analytically and creatively.
Context-setting
The act of designing is often referred to as problem-solving. However, as underscored by Hill in his book “Dark Matter and Trojan Horses,” this terminology often brings with it the risk of a narrowed perspective that could hinder creative exploration and innovation. This view often restricts the design scope to addressing only known-knowns or known-unknowns while excluding the potential for identifying underlying systemic issues.
The design process is far more intricate and unpredictable than merely solving problems. Often, designers question the original problem they set out to solve, realizing they may have tackled the wrong problem altogether. This line of thinking has led to the understanding that the term “problem-solving” might constrain the flexibility required to redefine the problem or challenge the original premise.
In this context, Hill further proposes “context-setting” as a condition for problem-solving. In his perspective, by shifting the focus from merely problem-solving to setting the context, designers can make a more holistic and broad-spectrum impact, considering the predetermined issues and the broader context and assumptions underlying those issues.
Building on this, it is worth considering Simon’s definition of design again, which frames the problem as an existing situation and the solution as a preferred situation. This definition provides a more flexible and broader perspective on design, emphasizing its transformative nature.
Frame innovation
The traditional discourse around “Design Thinking” typically emphasizes a designer’s capacity to produce solutions. However, an essential skill often overlooked is problem framing — the ability to conceptualize novel approaches to problem situations as outlined by Whitbeck in 1998. While valuable, the emphasis on solution generation can be seen as limiting because it implicitly operates within existing problem structures or “frames.”
In contrast, Frame Innovation brings to the forefront the necessity of shifting away from predetermined “frames” that have typically been conceived to function within reasonably isolated, static, and hierarchically ordered environments. The real world, however, is a dynamically interconnected system, which underscores the need for more systemic design approaches.
To delve further into the mechanics of problem-solving and design, we can refer to the typical basis for reasoning patterns in problem-solving, consisting of three components: “what” (elements), “how” (the pattern of relationships), and the “outcome” (observed phenomenon). These concepts are essential to various reasoning processes, such as deduction, induction, and abduction, adapted from Dorst’s work.
The critical difference in design thinking emerges in what we term “design abduction.” Unlike normal abduction, which starts with an observed phenomenon and works its way back to the elements and their relationships, design abduction begins with the known outcome and works backward within a particular frame. The choice of frame inherently involves certain assumptions and thus builds the ground basis for generating a preferred situation.
It is less about generating solutions and more about creating a bridge between existing and preferred situations. This critical shift underscores the idea that the creative moment in design is about establishing a connection between existing and preferred situations rather than the mere generation of solutions. This process can be done by iteratively adjusting the focus and finding the right frame.
In this perspective, the “frame” does not merely concern itself with what can solve a problem but also how it can be solved, thus creating a bridge between problem and solution space. However, framing is still intricately tied to our perception of the environment and the assumptions we bring into the design process.
Creative abilities of AI
Based on the model of Frame Innovation and the theoretical introduction of how the Large Language Models work, we will now consider how artificial intelligence could assist in design processes.
In the book “Design und KI,” it is suggested that creative processes are often transferred into “systematically guided, often professionally operated and institutionally supported strategies” to be consistently applied. In design, this expresses itself primarily through the rise of various frameworks and methods. It is argued that machines, especially AI, can participate in such processes, as they can operate systematically and methodologically. The underlying assumption is to understand creativity as a methodological approach rather than a serendipitous moment. As such, intelligent systems can indeed be creative, creating valuable outcomes, according to Engenhart and Löwe.
Nevertheless, it is crucial to remember that a machine has no intrinsic creativity as they outline. It is the executive power, driven and designed by human intention. The reflections on this point suggest that, regarding problem-solving strategies in Frame Innovation, AI still needs to possess the will or power of decision-making over the outcome of the design process.
Engenhart and Löwe conclude with an interesting proposition: viewing AI as an orthosis that enables designers to converge cognitively complex issues in design. Seeing AI as a tool that aids and enhances the design process rather than replacing human creativity was one of the guiding themes of this work.
System-oriented design
“Systems-Oriented Design,” as elucidated in the book “Designing Complexity” by Sevaldson, underscores the intrinsic interconnectedness and continual evolution of complex systems shaped by their components and environmental interactions. Emphasizing a perspective that surpasses a narrow focus on isolated elements, Systems Oriented Design points out that complexity is not a static feature but a dynamic characteristic that unfolds over time. This approach underlines the reality that complex systems are much more than the simple sum of their parts, producing unique and more significant outcomes than any single component could generate in isolation.
A key aspect of Systems-Oriented Design lies in its focus on adaptability and change. According to the principles outlined in the book, complex systems inherently adapt to their environments and evolve. Particularly in social systems, these complex entities frequently alter their rules, revealing an intricate web of interactions that we, as human observers, struggle to comprehend due to our cognitive limitations. This complexity often reduces systems to simpler conceptual objects, such as archetypes, clichés, and schemas, for us to make sense of them.
The book further clarifies that understanding a system only sometimes requires knowledge of all its components. Instead, it encourages comprehension as the “Gestalt” of systems. It warns against the potential danger of addressing only the symptoms of a system, as this could worsen the situation, given the complex interplay between the various elements within the system.
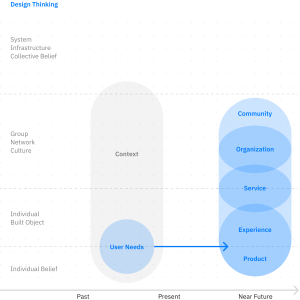
Masaki Iwabuchi outlines in his Medium article, how the previously introduced Design Thinking process works. Here, user needs are considered in a context and translated via the five-step process into a “solution,” which can take on different dimensions.
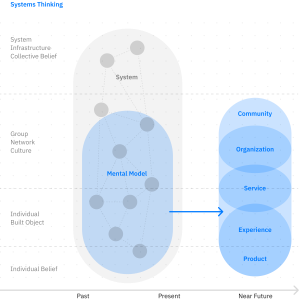
In contrast, the Systems Thinking illustration shows how the problem space can be addressed. It leaves out distinct groups of interest and focuses on understanding the dynamics between the system’s entities. Iwabuchi also introduces the concept of the mental model, which circumscribes the comprehension of the situation and highly depends on the observer of the system. As such, the mental model covers only a particular part of the investigated system since our perception is limited to abstraction.
Mental models
As we understand them, mental models are cognitive frameworks that provide a simplified representation of how the world operates. These frameworks underlie our beliefs and perceptions, thereby shaping our behavior and influencing our approach toward problem-solving and task execution. Derived not from facts but from individual or collective beliefs, mental models embody what users know, or perceive they know, about a system. As seen in the graphic above, some of these beliefs are in the conscious mind, but the majority are in the subconscious mind. Importantly, they possess a dual nature; they can either promote growth and innovation or hold back the same, contingent on their application.
In her work on the Doughnut Economy, Kate Raworth amplifies this perspective on mental models. She articulates that these models not only shape our understanding of the world but can also limit it. Quoting the systems thinker John Sterman, she mentions,
The most important assumptions of a model are not in the equations, but what is not in them; not in the documentation, but unstated; not in the variables on the computer screen, but in the blank spaces around them. Kate Raworth
In essence, the challenge with mental models arises from what they do not account for, thereby making certain aspects of our world invisible leading us to another fundamental concept in the perception of problem spaces.
Unknown unknowns
Unknown Unknowns refer to the unpredictable elements of a situation, those outcomes, events, circumstances, or consequences that are impossible to predict or plan for. It first gained widespread attention from former United States Secretary of Defense Donald Rumsfeld in a news briefing in 2002.
Unknown Unknowns play an immensely crucial role in design. They represent factors within the problem space that remain undiscovered until our ideas undergo testing. In interviews with the designers, the concept was often associated with the chaos and uncertainty encountered, particularly during the early stages of the design process. This insight may indicate that the experienced uncertainty and confusion can be partially attributed to the “Unknown Unknowns.”
Assumptions in Design
As an integral part of the design process, assumptions and biases can influence the course and outcome of the design. Cognitive biases, defined as systematic patterns of deviation from rational judgment, can affect decision-making, problem-solving, and, subsequently, innovation outcomes.
Jeanne Liedtka’s work is a good source in this regard, particularly her exploration of how Design Thinking can enhance the results of innovation processes. Her focus on cognitive biases and their impact on decision-making processes offers a comprehensive insight into the issue. Liedtka identified nine biases that can harm decision-making within the design process and summarized these in the table shown above.
In her conclusion, she articulates,
Humans often project their worldview onto others, limit the options considered, and ignore disconfirming data. They tend toward overconfidence in their predictions, regularly terminate the search process prematurely, and become overinvested in their early solutions — all of which impair the quality of hypothesis generation and testing. Jeanne Liedtka
Our investigation into design has identified several concepts, such as mental models, unknown unknowns, and assumptions, as bottlenecks for innovation. The primary objective of this article is to explore how to expand upon current design methodologies and frameworks and address earlier-mentioned innovation bottlenecks.
Nohr and Kaldrack, as introduced before, propose that speculation, as an analytical-narrative method, promises to disrupt one’s thought patterns, challenge basic assumptions and blind spots across knowledge regimes, explore hidden genealogies of the present, and envision new futures. This process, however, occurs under the condition of one’s entanglement in power and knowledge regimes, as well as an understanding of their origins.
This perspective aligns with a quote from Albert Einstein:
We cannot solve problems with the same thinking that we created them with. Albert Einstein
The quote encapsulates the objective of this work — the need to challenge assumptions and biases in design thinking processes and innovate beyond them.
Summing up
The exploration of various competencies integral to the design process, as discussed in the preceding sections, underlines that design is not merely a problem-solving exercise but a complex process involving identifying, framing, and redefining problems, followed by creating solutions. This process is influenced by various factors, including the designers’ mental models, assumptions, biases, and their ability to handle the “unknown unknowns.”
The concept of Frame Innovation brings to light the necessity of shifting away from established frames. It suggests that the creative moment in design lies in establishing a connection between existing and preferred situations. However, reframing problems is deeply tied to our perceptions and the assumptions we bring into the design process.
Artificial Intelligence’s creative abilities, systematic approach, and capacity for deductive thinking can enhance the design process. However, it is crucial to remember that AI, at its core, is a tool driven by human intention and thus lacks intrinsic creativity.
The discussion on Systems-Oriented Design underscores the importance of adaptability and change in addressing complex systems. It emphasizes the need for a comprehensive understanding of the system as a whole, warning against the potential dangers of addressing only the symptoms of a system and leading to the exploration of mental models, assumptions, and biases in design. These factors can limit our understanding of the world and hinder innovation if not adequately addressed.
In conclusion, the need to challenge assumptions and biases in design thinking processes and innovate beyond them becomes more apparent.
Risks of AI in design
In one of John Maeda’s experiments, he gives us a glimpse into a possible future of the design work. In one of his Python notebooks, he demonstrates AI’s capability to partake in the first three phases of the Design Thinking process. In the empathize phase, an LLM inputs a customer support log to generate sentiments and summarize the issues mentioned. These insights are used in the define phase, where the AI states specific customer problems. During the ideate phase, the semantic kernel, without any human intervention, brainstorms solutions to alleviate the customers’ pain points. These solutions are then categorized into lower-hanging fruits and higher-hanging fruits.
The power of large language models is undeniably evident, particularly in their ability to automate the conceptual part of the design process. This efficiency boost may appear very tempting; however, while beneficial, it can also be harmful. This risk is an important point that will be explored next.
AI as designer
Artificial intelligence can drastically transform our thinking and operation by augmenting the design process. However, one of the fundamental issues remains the inherent biases and probabilistic functioning of AI.
These biases result from AI only approximating the training data it provides. The first problem arises because the large language models are always only an approximation of the data and are unlikely to represent 100% of the data’s complexity. What happens when there are gaps in the data or the knowledge is not approximated accurately and therefore produces hallucinations?
While these hallucinations allow the generalizability of LLM’s capabilities, they can be incorrect and exaggerated. AI, trained to provide satisfying and user-friendly answers, can hallucinate such missing information, leading to biased results, and the AI’s suggestion that this information is accurate.
Moreover, the biases arise from the data and the assumptions upon which the AI was trained. This data only represents human perceptions and interpretations, as explained in “Preferable Futures”. It notes that “data-driven AI exposes the prejudices and wishful thinking of those who feed it, thus stabilizing social structures and expectations.” AI has been used in various contexts to limit uncertainties, whether in decision-making systems, training simulations, or full enterprise simulations. These applications share a common goal: controlling or making contingency controllable, leading to a rationality of “predictability.”
They further state that business and economic simulations aim to mitigate uncertainties while simultaneously programming how our society functions. It is not only about predicting futures scientifically but suggesting, directing, manipulating, and designing futures based on a belief in continuity, thus stabilizing trajectories and path dependencies.
This manifestation of thought structures becomes even more apparent with the image of self-reinforcing feedback loops on the right page. In these loops, an action influences the environment in such a way that this action becomes more and thus develops into positive or negative loops. Activities that lead to loops with harmful effects must be recognized.
This behavior manifests itself, particularly in the thought structures, which in turn have an effect on behavior in societies. If, for example, we think that the car is the best means of transport, new governments build more roads based on this general assumption. The advanced development of road infrastructure also leads new generations to think that a car is a practical means of transport. Our actions, therefore, solidify the initial thought structures.
If we aspire to implement more profound societal changes, we must question our thought structures to tackle climate change or species extinction. In the book “Transformationsdesign,” the authors refer to “change by design or change by disaster.” As Sommer and Welzer further state, socio-ecological transformations involve external conditions and people’s perceptions, self-images, emotions, and habits. Therefore, changes in these cultural-mental formations must primarily occur in practice, not just in cognitive work.
To summarize these complex matters more simply, there is a quote by Rolf F. Nohr:
Or to put it more bluntly: the (uncertain) future imploded into a kind of “feedback-effected present” in which tendencies are intensified or subdued. The future was hedged and immobilized Rolf F. Nohr
The fundamental idea is that we have no absolute independence of action since our decisions are based on the present thought structures.
The requirements to act based on new thought structures arise from the will to create a wide-ranging preferred situation. However, as seen before, large language models are built based on data, which maintains the fixed thought structures of our society. From this emerges that artificial intelligence cannot solve problems that require a change of thinking structure. A further issue is self-reinforcing loops, with which artificial intelligence continues reinforcing the thought structures built into it, as described in “Preferable Futures.”
It becomes clear that the current limitations of AI rest heavily on its inbuilt structures, shaped by existing human knowledge and prejudices, which cause replication of our societal biases, sometimes even amplification. This issue extends to the core of AI-driven applications aiming to control or foresee uncertainties.
These self-reinforcing feedback loops further solidify these societal thought structures, influencing actions and expectations that drive our societies. Consequently, these feedback loops reinforce existing systems and potentially increase societal norms. The demand for a more comprehensive societal transformation, as necessitated by crises like climate change and species extinction, requires a reassessment of these profoundly ingrained thought structures.
Thus, despite its considerable promise, AI, as it stands, may struggle to address challenges that require a paradigm shift, mainly because it operates within and potentially accelerates the established thought structures of our society. Future discussions should consider this critical aspect while evaluating the utility and potential of AI-driven solutions.
Augmentation of the design process
In recent years, the conversation around design processes has turned increasingly toward adopting artificial intelligence and automation. This article aims to revisit the ideas of thought leaders such as Doug Engelbart and clarify why augmentation, not automation, is vital for the design processes. This consideration is underpinned by a critical intent-leveraging design to solve intricate yet meaningful problems.
We discovered that Design Thinking had driven a shift towards considering broader environmental factors. Additionally, frameworks like Futures Thinking underscored the importance of envisioning new potential futures. Drawing on the Lean UX approach, we also learned to work based on assumptions to manage the uncertainty inherent in the design process.
In the quest for problem-solving, a novel definition was found in Frame Innovation, focusing on how designers transition from current to preferred situations. We evaluated the capabilities of AI in this context and further delved into the topics of mental models, unknown unknowns, biases, and assumptions within the design process. All these factors, significantly when poorly managed, can adversely impact the design process results.
Therein lies the contradiction of the intent. Automation in design, primarily through AI, comes with its risks. AI might reinforce existing biases and influence decision-making, as it depends heavily on the data it is trained on. It needs a deeper understanding of context, intuition, and emotion. Moreover, in cases where data is absent, AI might “hallucinate,” leading to even more concerning outcomes.
The investigation concludes that while AI has its role, it should be seen as an augmentative rather than an automated one. Due to entrenched thinking patterns, this approach prevents the future from becoming “hedged and immobilized” as found out before. It allows us to maintain the design process’s complex, nuanced, and deeply human aspects.
The thesis iteratively explored potential use cases for large language models that amplify the augmentation of the design process without influencing the design outcome. The generative capabilities of artificial intelligence were found to be advantageous for the design process. However, it was also recognized that data generation could again lead to increased complexity in the design process. Thus, the thesis aimed for a solution that, despite its generative and divergent capabilities, reduces the complexity of the design process.
Furthermore, AI should not make decisions or generate ideas that can influence the designer, leading to the use case of AI stimulating reflection in designers for mitigating uncertainty in the design process.
The use case of stimulating reflection in designers emerged through an exploratory approach. In this context, designers’ ideas or assumptions should be contemplated in collaboration with large language models. The intention behind this is to identify gaps in the design argument that can arise due to the mental models, assumptions, and biases of the designers. The broader goal of the application is the discovery of “unknown unknowns,” which the designer inherently cannot recognize alone.
The responsible use of AI is thus ensured by not generating decisions or ideas but by providing stimuli for reflection that go beyond what the designer could consider unaugmented. This augmentation allows for a broadening perspective, enabling designers to challenge their inherent biases and assumptions. Reflection and questioning interrupt the manifested self-reinforcing feedback loops by exposing and questioning underlying assumptions before decision-making.
In essence, the augmentation of design processes through AI does not entail giving decision-making power to AI. Instead, it is about harnessing the generative capabilities of AI to help designers identify their biases and assumptions and illuminate “unknown unknowns.”
The focus is to reduce the complexity of the design process, not by filtering out valuable information but by enabling designers to manage uncertainty better, enhancing their capacity to transition from existing situations to preferred ones.
This approach aims to ensure that AI is implemented as an effective tool for augmenting the design process, providing stimuli for deeper reflection, and challenging existing biases and assumptions. By doing so, AI can play a positive and sustainable role in future design processes, not by seizing human designers but by augmenting their abilities and expanding their perspectives.
Related works
Upon finding a conceptual framework that underlines the augmentation of the design process through AI, it was crucial to examine practical applications and related research that embody these principles. One such exploration is the development and implementation of systems that leverage AI to enhance understanding and interaction with complex information and support human reflexive processes.
Graphologue
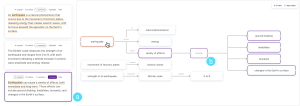
The paper Graphologue presents a novel interactive system called Graphologue, designed to convert text-based responses from Large Language Models like ChatGPT into graphical diagrams. The motivation for this system comes from the identified limitations of text-based mediums and their linear conversational structures, which can make it challenging for users to comprehend and interact with complex information.
Graphologue employs prompting techniques and interface designs to extract entities and relationships from LLM responses, constructing node-link diagrams in real time. Users can interact with these diagrams to adjust their presentation and submit context-specific prompts for more information. This facilitates a visual dialogue between humans and LLMs, enhancing information comprehension.
Graphologue allows the users to control the complexity of the diagrams, enabling them to toggle between sub-diagrams to show salient relationships, collapse branches of the charts to reduce presented information, and combine separate smaller graphs into one diagram to view all concepts as a whole. Users can also directly manipulate the graphical interface, translated into context-aware prompts for the LLM.
Especially at the beginning of the topic identification, the complexity and interconnectedness of the contexts in which we design played a significant role in the work. Initial hypotheses the interviews were led with similar opportunities for visually processing information.
Riff
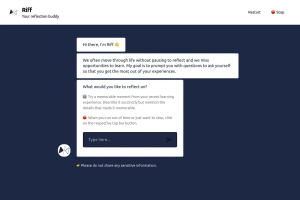
The article “Reflecting with AI” by Leticia Britos Cavagnaro discusses developing and using an AI tool called Riff for enhancing reflection. Riff is a conversational AI chatbot that uses a GPT 3.5 Large Language Model to generate dynamic questions based on user input, encouraging learners to delve deeper into their experiences.
Riff’s primary purpose is to augment individual reflection. It achieves this by asking questions that prompt the learner to elaborate on their experiences, contrast their current experiences with past or hypothetical ones, explore beyond their initial observations, and consider how their future actions might change based on their reflections.
Riff differs from other AI tools like ChatGPT in its interaction style. While users typically ask ChatGPT questions or request it to perform tasks, Riff asks users questions and continues to ask follow-up questions as they respond. This approach is intended to create an engaging conversation that should lead to reflection.
Riff’s interaction with the user will be an important parallel to this article. The use case is not meant to provide the user with answers or inspiration but to ask more profound questions encouraging them to reflect on their assumptions.
Transitioning from the examination of related works for the AI’s role in enhancing user interaction and reflection through Graphologue and Riff, the discussion shifts towards the creative and experimental side of AI applications in design. This pivot underscores the exploration of unconventional approaches to utilizing AI, aiming to provoke thought and inspire innovation.
Sacrificial concepts
Several rationales were identified in supporting designers through the use of LLMs for mitigating uncertainties in the design process. Sacrificial concepts were developed to make this process tangible and open for discussion by exaggerating various ideas intentionally. This approach ignored the feasibility and economic viability boundaries to attribute almost magical qualities to the different archetypes.
The concepts implicitly present negative aspects and consequences of AI applications. The intent was to test how the interviewees perceived these nearly magical promises and where they started to show skepticism. Still, it also aimed to understand what negative consequences they were already aware of and could recognize.
Through interviews with experts, it was possible to collaboratively evaluate which negative attributes significantly impacted the design process or society and which had lesser consequences. This evaluation was critical in understanding the trade-offs when implementing AI in design processes.
The map
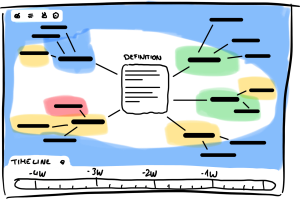
The first concept, “the map,” symbolizes a comprehensive guide that helps designers traverse the often ambiguous landscape of a project. Like a geographical map, it shows the uncovered areas representing the unexplored or unfamiliar aspects of the project. It provides orientation by depicting the overall context within the design process. This context might include the project’s objectives, constraints, stakeholders, and dependencies.
A critical aspect of this map is that it’s not a static entity. It is constantly updated, reflecting the evolving nature of the project. As new information is uncovered and the context changes, the map is revised to remain current and relevant. The map is a single source of truth, meaning it can be relied upon for accurate and up-to-date information. In the face of uncertainty, it offers guidance by helping designers visualize the bigger picture, understand their current position, and determine their directions.
The dangers presented by this concept lay primarily in the suggested completeness that a map should otherwise provide. However, this exhaustiveness is impossible in uncertainty and cannot be provided by the AI application, as shown before.
The magnifying glass
The second concept, “the magnifying glass,” represents a tool that aids designers in scrutinizing the details of their work. Picture a designer with many post-its and notes — these often contain various assumptions and ideas. The magnifying glass metaphorically “glows” at unvalidated beliefs, making them apparent. This allows designers to recognize when a hypothesis hasn’t been tested or validated.
Moreover, looking closely through this magnifying glass, all the risks associated with these assumptions become visible. It guides the designer’s attention to areas that require reflection and critical analysis. This is particularly valuable because it is easy to overlook the nuances and risks of certain decisions in the excitement of creative processes.
The difficulties indicated here are that the tool itself cannot understand the effects and implications of the assumptions and, therefore, cannot give a valuation. The evaluation is also problematic, as will be seen more strongly in the next concept.
The search dog
The third concept, “the search dog,” embodies a guide that helps designers find the right path through their projects. Like a rescue mission search dog, it has an innate sense of direction and can see what’s important. It highlights the assumptions that should be the focus and pulls the designer through the project.
This concept represents a source of strong guidance. Unlike the map, which provides a broader orientation, or the magnifying glass, which focuses on detail, the search dog offers operational advice. It helps determine which aspects of the project are crucial and should be prioritized.
The dangers of this concept lie in the automatic scoring and prioritization of tasks. This could lead the user into a dependency that makes it easier to be guided through the search dog despite the designer’s ability to intervene.
Outcomes
While analyzing the outcomes of the interviews regarding the sacrificial concepts, several key insights and reflections emerged. The participants recognized and highlighted some potential dangers and the usefulness of the sacrificial concepts.
Firstly, “the map” was acknowledged as an effective tool, but some participants were apprehensive about its potential to create dependency. Similar to how individuals rely on Google Maps for navigation, there was a concern that designers could become overly dependent on “the map” for orientation. This reliance could hinder designers’ ability to think independently or creatively without the tool. However, despite this concern participants found “the map” tangible and usable. The metaphor of the map guiding one through a path of uncertainty was well-received, as it depicted the map’s ability to provide clarity and direction amidst the complex landscape of a design project.
Next, “the magnifying glass,” with its glowing assumptions, was considered particularly intriguing by the participants. They found value in its ability to highlight vague ideas, as these could be more innovative. The magnifying glass’s capacity to draw attention to assumptions and risks was seen as empowering, allowing designers to evaluate and refine their ideas critically.
Regarding “the search dog,” participants noted its representation of automation in the design process. However, this was a double-edged sword. While the search dog was seen as powerful due to its ability to see and sense things that might not be immediately perceptible to humans, there was criticism regarding the lack of genuine participation in the design process. The automation that the search dog symbolizes could potentially alienate designers from the hands-on aspects of their work.
One overarching theme that became particularly prominent through the development and discussion of the sacrificial concepts was the influence of these systems on the designers themselves. The way these tools might shape not just the design output, but the thinking and working patterns of the designers, was a recurring topic. This aspect was crucial and was considered throughout the further development of the concepts.
The discussions surrounding the sacrificial concepts not only illuminated their potential applications and limitations but also sparked a deeper reflection on the nature of design tools and their impact on creative processes. As we transition from these insights into the specific functionalities of the proposed system, it becomes apparent that the intention behind this design philosophy is to foster an environment of dynamic interaction and adaptation. This approach aims to empower designers to engage more deeply with their work, encouraging a blend of creativity and critical thinking.
To make the results of the previous investigation more tangible, the design rationale and decisions for the concepts are elaborated below.
Concept
Within each project, all ideas and assumptions are intended to be placed on a canvas. This design choice aims to render the information visually and functionally easily manipulable. By arranging data and ideas on a canvas, users can more effectively interact with the knowledge, modify it, and perceive it as adaptable rather than fixed.
The application employs overarching panels for information relevant across the entire project, such as the description of objectives, constraints, and the description and customization of the language model. This hierarchy supports and visualizes the cross-project impact and manipulability by the user. It ensures that critical information across the entire project is easily accessible and can be altered for the user’s requirements.
Another feature regarding the nodes is that information within a node can be directly adjusted. This includes toggling the node’s status via a single click at the marker. Such a feature enhances the user’s interaction and control over the data points. The text on the node can also be adjusted by clicking the text field.
Furthermore, users can directly provide feedback on the generated assumptions on the node. This function is positioned next to the author “anticipate,” which helps in clarifying that the feedback is meant for the language model.
Additionally, nodes can be deleted but remain existent even after deletion in the hidden view. This feature is significant as it ensures that users retain valuable information and can retrieve it when necessary. Furthermore, they can be used as a negative prompt that allows the LLM to further align with human intent.
Mental model
The testing of different stages has revealed numerous challenges in the application, resulting in the mental model’s rework. Initially, the term “assumption” was mainly used; however, it was found that this term is not commonly employed and posed difficulties for even skilled designers in formulating their assumptions.
For this reason, the initial assumption was rephrased as “idea” as it proved more accessible for various groups to be expressed. Typically, the idea is documented once the objective (goal) and constraints of the action scope are defined. This approach is intended to simplify the articulation of ideas and create a coherent overall picture for the language model.
Subsequently, the AI generates presumptions based on the formulated idea, which the user can validate for the concept to work effectively. Here, the term “generate” is used to highlight the contribution of the language model, while the instruction to type signifies the possibility of manual input from the user.
Views
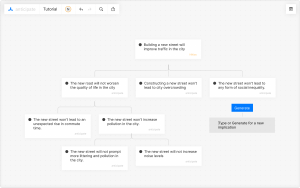
The concept incorporates views and a tree structure to establish a hierarchical organization of assumptions like a decision tree. The initial ideas are distinctly visible and navigable on the canvas. As one explores these ideas, the increasing level of detail in the tree’s nodes becomes apparent and easily navigable.
Efficient navigation is facilitated on the canvas, with significant space efficiency simultaneously due to the compressed but flexible trees. This layout yields a clear overview, benefiting newcomers and aiding in the tree’s construction, as one can navigate from top to bottom through the tree.
Employing tree structures as a medium is prevalent for making decisions or organizing thoughts, making this highly relevant for practical application. The tree branches can be easily minimized or hidden without affecting the functionality of the tree.
In addition, custom views can be created for personal mental integration of assumptions into the design process. These adjustable views may include matrices where nodes can be sorted and prioritized according to individual metrics.
Furthermore, different visibility options for nodes enable users to hide or show nodes they have created, nodes generated by the AI, or nodes that have been deleted. This flexibility in managing visibility supports a more tailored approach to organizing and analyzing the information within the tree structure.
Project description
In the concept, the project description is pivotal in providing artificial intelligence with the necessary context for generating assumptions. Initially, this contextual description was general and could encompass any information. However, it was later understood that the context description could also assist users in capturing their intentions, making it easier to respond with an initial idea.
Furthermore, the general field was divided into two segments: objectives, which represent the goals the project aims to achieve, and constraints, which mark the boundaries of what is feasible within the project. The purpose of defining these constraints is to prevent the AI from suggesting options that are not relevant to the project.
A critical aspect of this process was finding the right balance between providing guidance for valuable results and keeping the outcomes open-ended. This balance is essential not to confirm pre-existing notions but to allow unexpected and surprising elements to emerge.
AI explainability
The AI Panel is a feature that allows users to adjust their expectations of the AI’s capabilities through detailed explanations of the inner workings. This is also accomplished through a control board that changes the functionalities of large language models to the specific purpose. The AI Panel aims to make the influence of implicit feedback more transparent and understandable to the user.
One of the key features is the option to experiment with various AI models, which aids in achieving cost efficiency. Further experimentation is facilitated through sliders that encourage users to guide the AI-induced reflections in a desirable direction. Moreover, explanations for each slider, including information on their significance, impact, and functionality, are provided to offer users a thorough understanding of how the sliders operate.
Additionally, users can define their objectives at the bottom of the AI Panel. This feature is designed to influence the AI’s behavior and responses directly.
The AI Panel is supplemented with detailed descriptions for all parameters. This is intended to enhance transparency and allow users to comprehend better the workings and implications of the various elements within the panel. Through these features, the AI Panel enables users to have more control and customization in their interaction with the AI, adapting it to suit their specific needs and goals.
Classifications
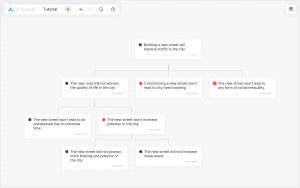
The concept incorporates a system of categorizing assumptions through markers. Initially, assumptions were marked as default, validated, critical, or irrelevant. However, experiences drawn from utilizing the prototype indicated the necessity for alterations. Default and validated categories were merged, as a validated assumption was found to have an equivalent level of influence on the idea as a default node. In both instances, it is presumed that the idea remains validated.
Furthermore, the irrelevant marker evolved into deleting the node, where only hidden nodes remain accessible under a specific view. This adaptation is intended to foster a more streamlined and organized tree structure. Additionally, this alteration ensures the retention of nodes that would otherwise be discarded, and deeming them irrelevant becomes a more traceable decision.
This reclassification of assumptions is designed to optimize the clarity and organization of the tree while preserving the critical elements that contribute to the evolution and functionality of the idea.
Collaboration
The insights gathered from interviews identified a substantial opportunity for improvement in collaboration with stakeholders. Uncertainties often challenge this collaboration, and the tool aims to alleviate this issue by facilitating stakeholders in articulating their diverse ideas and discussing the associated presumptions and implications.
By enabling this, the concept fosters collaboration among stakeholders from various backgrounds. It creates an environment where ideas can be discussed more openly and sincerely. This, in turn, contributes to a more efficient and cohesive decision-making process, allowing for the collective input and expertise of all stakeholders involved.
Prototype
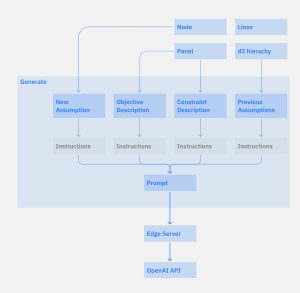
The primary purpose of the prototype was to validate the concepts functionally and make the idea more accessible to explain and test together with others.
Users could input their ideas and evaluate whether the results were relevant to their thought processes. This immediate interaction and feedback were beneficial in understanding and adapting to user needs and expectations. There was also a distinct shift in the discourse regarding the content of the approach. The applicability of the results became more verifiable, allowing for a more in-depth and meaningful discussion.
The insights gained through the prototype were in a favorable ratio concerning the time invested in its development. Although there was no previous knowledge of developing such a prototype, the implementation was smooth, with only minor problems. It provided valuable information without requiring excessive time in its creation.
The prototype presents an opportunity for further enhancement, primarily through integrating findings from the development of the interface. This integration would yield additional insights into its usability and can further optimize the prototype.
Moreover, it would be exciting to continue examining the utilization of language models. In this context, it would be beneficial to fine-tune a large language model to refine the results further. The fine-tuning process would allow the language model to be more aligned and calibrated with the specific requirements and objectives of the application.
Additionally, a more intensive examination of the prompts would be advantageous in improving the results of the functional prototype. A thorough evaluation and optimization of the prompts can ensure that the language model receives the necessary information and context, resulting in more relevant and accurate responses.
The prototype can be tested here: prototype.anticipate.studio
Conclusion
This exploration stemmed from a genuine curiosity about artificial intelligence, its capabilities, and more importantly, its implications for design and society. Deeply influenced by my personal interests and the culmination of my design education, my work has been an attempt to integrate technological advances with human-centered principles.
The essence of my thesis revolves around a critical perspective on the emerging role of AI in design processes. In an era where the temptation of automation is undeniable, my research underscores the need for a balanced approach — augmentation over automation. This distinction is critical, not only to preserve the integrity of design thinking but also to ensure that AI serves as an ally to human creativity rather than a replacement.
In addition, the thesis explored the inherent biases of AI, a reflection of its training data and, by extension, our societal biases. This exploration was not an indictment of AI, but a call to action to develop systems that are aware of these biases and equipped to challenge them.
In synthesizing the findings from the experiments and the broader discourse on AI in design, it becomes clear that the journey towards integrating AI into design processes is fraught with challenges, but also full of opportunities. The thesis articulates a vision in which AI does not dictate design outcomes, but rather stimulates reflection, enabling designers to uncover “unknown unknowns” and challenge their assumptions. This approach is not simply about mitigating the risks associated with AI, but about using its generative capabilities to enrich the design process.
In conclusion, this thesis has been as much a personal journey as it has been an academic one. It has been a process of self-reflection, a means to process my learnings and experiences, and a way to envision a future where AI and human creativity come together to foster a more thoughtful, inclusive, and nuanced design practice.
The exploration underscored the importance of maintaining human agency in the age of artificial intelligence, advocating for systems that augment rather than automate, that question rather than conform. As we stand at the intersection of technological advancement and human-centered design, I believe the path forward is one of collaboration, critical thinking, and continuous reflection.
Following the thesis, I began researching with Aeneas Stankowski on the designability and discoverability of reflective capabilities in LLMs. This expanded to a collaboration with Valdemar Danry of the Fluid Interfaces group at the MIT Media Lab to explore how language models can augment human cognitive abilities, particularly in navigating the complexities of social media content.
Big Idea Initiative is all about making connections, and sharing knowledge, thoughts, and ideas that support deep thinking and collaboration. Our goal is to create a space that sparks thinking and conversations among people whose ideas might benefit each other, even if they’re working on completely unrelated topics. We think that pushing back the limits of possibility will come as a result of the connections that diverse collaborators make together. Identifying these connections will bring the big ideas our world needs.
We need your help! If you…
- have questions or feedback about this work
- want to improve, develop, or add to this idea
- want to sponsor a prototype of this idea
we invite you to contact us: hello@bigideainitiative.org.